The rapid advancement of AI technology has brought about numerous benefits and opportunities. However, it has also raised ethical concerns and highlighted the need for a robust ethical framework to guide its development and implementation. In order to ensure responsible and accountable AI systems, it is crucial to emphasize the importance of a technology-neutral ethics that considers both individual and collective aspects.
Programmers play a crucial role in ensuring that AI systems are designed and implemented in a way that aligns with ethical principles and values.
Furthermore, It is crucial to involve diverse stakeholders and ensure transparency in the development and deployment of AI systems. This helps to build trust and accountability, as well as address potential biases and discriminatory outcomes.
Additionally, the appearance of foundation models (AI models designed to produce wide range of tasks) comes with numerous benefits, such as the ability to perform complex tasks and increase productivity. However, they also come with risks that need to be carefully addressed with an ethical framework.
In conclusion, the need for an ethical framework in AI is essential. It provides guidance and principles for the implementation and deployment of AI systems, ensuring that they are responsible, accountable, and aligned with societal values.
Responsible AI
Responsible AI is not just a fancy term; it's a call to take action.
At Responsible AI from principles to practice, Virginia Dignum suggest to consider AI not only as an automation technique, instead AI is much more, is a socio-technical ecosystem, recognizing the interaction with technology, and how it is affected by humans behavior and society.
It highlights the ethical, legal, and social factors that come with the development, deployment, and use of AI systems. Responsible AI does not mean that the system that we create is responsible, it represents a dedication to ensuring that AI technologies are used responsibly, with transparency, and with a thorough understanding of their impact on society.
Virginia, defines the ART principles (Accountability, responsibility and Transparency). Accountability in AI refers to the system's responsibility to provide explanations and justifications for its decisions to users. The concept of responsibility emphasizes the role of individuals in their interaction with AI, while transparency refers to the system's ability to describe, inspect, and reproduce the process used by AI systems.
In order to achieve Responsible AI according to IBM, it is important to consider:
- Fairness: The presence of bias in input data can lead to unfair or discriminatory outcomes. Ensuring fairness in AI models is crucial, as bias can inadvertently encode discrimination into automated decisions.
- Data Laws: Legal restrictions on data usage and sharing pose challenges, making it essential to comply with data regulations while developing AI systems.
- Transparency: Ethical AI systems must be transparent about the data they collect and how they intend to use it.
- Privacy & Security: Privacy concerns, especially related to sensitive information, need to be addressed.
- Value Alignment: AI systems should align with human values and ethical principles to avoid generating false or harmful content, such as hallucinated information.
- Misuse: AI systems can be misused to generate misleading, toxic, or abusive content, posing risks to individuals and society.
AI Ethical Frameworks
There are many AI Ethical Frameworks that provide recommendations to follow. As mentions UNESCO, an AI Ethical Framework should prioritize proportionality, avoiding harm, and respecting human rights. It should emphasize safety, fairness, non-discrimination, minimizing bias, equitable access, sustainability, and privacy. Human oversight, control, and accountability are essential, along with transparency, explainability, responsibility measures, public awareness, and multi-stakeholder collaboration for inclusive AI governance.
It is good practice to define measurable indicators that provide insights and enable control throughout the lifetime of an AI system. For example, the Ethical Application of AI Index (EAAI) framework emphasizes several key aspects for ethical AI. These indicators are used to calculate an overall score and evaluate an AI system.
- Bias in AI: Owners of AI systems should address bias by understanding and monitoring how it influences AI inputs, algorithms, and interpretations. Collaboration and monitoring indicators such as accountability, diversity, and data bias can help ensure ethical AI application.
- Fairness in AI: AI systems should prioritize fairness by avoiding discriminatory outcomes and addressing potential risks such as data bias. This requires transparency, interpretability of algorithms, ongoing evaluation, and monitoring through testing, auditing, and user feedback. The goal is to minimize unfair advantages and achieve equitable results.
- Transparency in AI: AI systems should be auditable and explainable to decision-makers and the public. Transparency involves explaining how and why a system reaches a particular outcome, being open about algorithms used, and monitoring indicators like fairness and bias. Continuous monitoring, audits, and tracking performance are important for responsible and transparent AI application.
- Responsibility in AI: The responsible application of AI requires accountability, protecting stakeholders, and ensuring relevant, reliable, legal, authentic, auditable, and effective results.
- Interpretability in AI: Stakeholders should have a thorough understanding of AI systems, producing reliable and trustworthy results. Clear and consistent outputs that are understood by different stakeholders are crucial, along with monitoring and measuring indicators throughout the project lifecycle. The framework also recognizes the role of AI in informing and transforming perspectives based on past experiences and current circumstances.
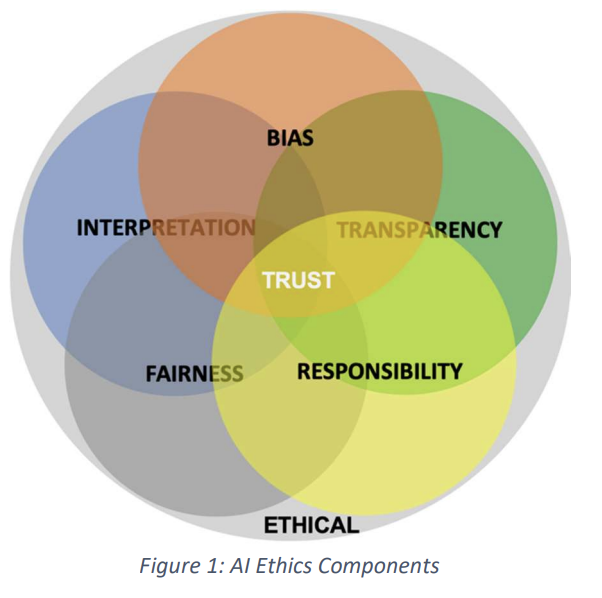
There is no general framework that fits all organizations. It depends on the context and the specific reality of each organization. You need to create a custom framework that adapts to your needs.
For example, Facebook uses Fairness Flow, a custom toolkit for analyzing how AI models and labels perform across different groups. Fairness Flow measures models based on their deviation from the labels they were trained on. It helps identify potential statistical biases and enables product teams to make informed decisions and take necessary steps to ensure fairness and inclusivity in AI systems.
Another example is Google, which utilizes custom explainable AI tools and frameworks to understand and interpret predictions made by machine learning models. This improves model performance and generates feature attributions. Google integrates these tools with its products and services, allowing for a better understanding and trust in AI outputs.
Model Cards
Model cards are a proposed framework to provide transparent and structured information about AI and machine learning models. They aim to address the lack of understanding regarding how these models function, including their strengths and limitations. These cards would cover a wide range of information, from the model's architecture to its performance under various conditions. Model Cards are standardized documentation formats used to provide transparent information about machine learning models. They were introduced by Google in 2018 to promote responsible AI development and accountability. Model Cards cover key aspects of a model, such as its details, architecture, training data, performance metrics, potential biases, and responsible AI considerations. Several companies have adopted Model Cards (AWS SageMaker, HuggingFace, Kaggle), and IBM introduced AI FactSheets, a similar concept for comprehensive model documentation. Model Cards are important for promoting transparency, informed decision-making, reducing bias and errors, facilitating collaboration, and ensuring responsible AI development.
Data Protection considerations
The Alan Turing Institute emphasizes the principles of fairness, transparency, and accountability outlined in the GDPR. Fairness involves providing explanations for AI-assisted decisions to ensure autonomy and self-determination. Transparency requires being open about how and why personal data is used, including the training and testing of AI systems. Accountability involves demonstrating compliance with GDPR principles, such as data minimization and accuracy, by providing explanations and documenting decisions. Important considerations include the right to be informed, the right of access, the right to object, and the need for Data Protection Impact Assessments. The Equality Act 2010 also applies to various organizations, prohibiting discriminatory behavior based on protected characteristics. Other regulations, such as the HIPAA Privacy Rule and CCPA, are required in specific areas or states.
These regulations and principles play a crucial role in ensuring the responsible and ethical use of AI and protecting individuals' rights and privacy. Compliance with these guidelines helps establish trust and accountability in AI systems, addressing concerns related to fairness, transparency, and data protection.
Privacy-Enhancing Technologies (PETs):
In the ever-evolving landscape of digital privacy, Privacy-Enhancing Technologies (PETs) emerge as guardians of sensitive information, employing advanced techniques rooted in cryptography and statistics. These technologies have clear objectives: ensuring secure access to private datasets, enabling cross-organizational analysis without compromising individual data security, fortifying cloud computing for private data, decentralizing user data-dependent systems, and processing data anonymously. PETs wield a diverse set of cutting-edge techniques, such as synthetic data generation, differential privacy, homomorphic encryption, secure multiparty computation, federated analysis, trusted execution environments, anonymization techniques, and cryptographic methods. Implementing PETs involves a strategic approach, including risk evaluation, proactive risk reduction measures, and the careful selection of PETs that align with the goals of minimizing, separating, concealing, and aggregating data. These strategies, whether data-oriented or process-oriented, pave the way for a privacy-centric digital future where innovation and data security coexist seamlessly.
Challenges in Ethical AI
As artificial intelligence continues to change our world, the ethical concerns related to AI have become a major topic of discussion. While AI brings impressive advancements, it also poses numerous challenges in terms of ethics. In this section, we will examine some of the most important challenges encountered in the field of Ethical AI. The challenges introduced by IBM are:
- Governance: Determining responsibility for misleading AI-generated output can be difficult, especially in complex scenarios involving multiple parties.
- Explainability: It can be challenging to explain why a particular output was generated by an AI model, as some models operate as "black boxes."
- Traceability: Identifying the original source of data used for training an AI model can be difficult, particularly when data is sourced from multiple sources.
- Legal Compliance - Intellectual Property: Issues related to intellectual property and copyright can arise when using data to train AI models.
Maximizing all dimensions at once can be challenging. For instance, prioritizing privacy may hinder the ability to explain model behavior, and enhancing transparency may introduce security and privacy risks. Furthermore, group fairness and individual fairness require different approaches and have their own limitations.
Moreover, when addressing ethical concerns in AI, it's essential to be aware of potential pitfalls and traps that can hinder ethical progress. These traps include:
- The Solutionism Trap: Relying solely on technological solutions without considering broader socio-economic challenges can lead to inadequate solutions.
- The Ripple Effect Trap: Introducing technology into social systems can have unintended consequences, altering behaviors, outcomes, and values.
- The Formalism Trap: Mathematical fairness metrics may not capture the complexity of fairness. Fairness is context-dependent and should be addressed on a case-by-case basis.
- The Portability Trap: Reusing AI models across different social contexts without considering differences can lead to misleading results and unintended consequences.
- The Framing Trap: Focusing solely on technical aspects of AI ethics without considering the broader social context can result in incomplete or ineffective ethical frameworks.
Ethical questions about Ethical AI
Here are some ethical questions that emerge from the concepts explained in paper ¨Machine Ethics: The Design and Governance of Ethical AI and Autonomous Systems¨.
How to test whether the models are ethical or not?
The Comparative Moral Turing Test (cMTT), a human interrogator judges morally significant actions performed by a human and an ethical machine. If the machine's actions are consistently judged as less moral, it passes the test. However, critics argue that this test may set the moral threshold too low. An alternative is the Ethical Turing Test (ETT), where a panel of ethicists evaluates the machine's ethical decisions across different domains. If a significant number of ethicists agree with the machine's decisions, it passes the test. But also the concept of an ethical Turing Test is a topic of controversy. Some people argue against it, primarily because the test treats the ethical agent as a black box, focusing solely on its decisions without considering the underlying reasoning. They advocate for a verification approach that prioritizes predictable, transparent, and justifiable decision-making and actions, a viewpoint with which the authors of this text strongly concur.
Can machines learn ethical principles?
Yes, but there is a problem called ¨the issue of learned ethical principles¨, which arises when machines acquire ethical principles from observed decisions rather than relying on pre-established principles. This approach is in line with the scientific study of ethics, which seeks to identify principles that can explain moral judgments based on the reasoning used to support them.
Who determines that it is ethical or not?
The Trolley Problem is an experiment often used to discuss the ethics of AI, especially in relation to driverless cars. There is a study ¨The Moral Machine¨ that presents a scenario where a driverless car has to choose between two bad outcomes, and you have to choose. The study, demonstrates that exists significant cultural variations in the answers. Therefore, an AI system might behave well for some people and not so well for others. Even if we manage to overcome the technical challenges, it is crucial for society to engage in a comprehensive debate to establish clear rules and protocols for machines when they encounter ethical dilemmas.
Recommendations
In conclusion, addressing ethical bias in AI is crucial for the responsible and inclusive development of AI technology. Big companies like Google and Facebook have provided valuable recommendations to mitigate bias in AI systems. These recommendations include promoting data diversity, implementing bias detection tools, fostering inclusive teams, ensuring fair user experiences, establishing feedback mechanisms, conducting ethical impact assessments, and collaborating with external experts for third-party audits. By following these recommendations, we can create AI technology that is more inclusive, fair, and beneficial for all users while reducing bias-related issues. It is our collective responsibility to prioritize ethical considerations and ensure that AI systems align with societal values and respect the rights of individuals.
Tools
Visualization
- https://github.com/pair-code/what-if-tool
- https://github.com/PAIR-code/facets
- https://github.com/uber/manifold
- https://github.com/Trusted-AI/AIF360
- https://github.com/DistrictDataLabs/yellowbrick
- https://github.com/fairlearn/fairlearn
Fairness during training
Interpretability
- https://github.com/tensorflow/lucid
- https://github.com/shap/shap
- https://github.com/andosa/treeinterpreter
- https://github.com/pytorch/captum
Transparency
Explainability
- https://github.com/SeldonIO/alibi
- https://github.com/Trusted-AI/AIX360
- https://github.com/marcotcr/anchor
- Tensorspace
- Microscope
- Amazon SageMaker Clarify
LLMs guardrails
- https://github.com/ShreyaR/guardrails
- https://github.com/NVIDIA/NeMo-Guardrails
- Fairlearn
- LLM-guard
Differential Privacy
Federated Learning
- https://github.com/OpenMined/PySyft
- https://github.com/facebookresearch/FLSim
- https://github.com/tensorflow/federated
Encryption
- https://github.com/facebookresearch/CrypTen
- https://github.com/mortendahl/tf-encrypted/
- https://github.com/google/fully-homomorphic-encryption
References
- AlgorithmWatch, Ethics and algorithmic processes for decision making and decision support
- IBM - Foundation models: Opportunities, risks and mitigations
- UNESCO - Recommendations
- ACT-IAC Emerging Technology COI, White Paper: Ethical Application of AI Framework, (2020)
- Google Responsible AI
- Facebook’s five pillars of Responsible AI
- Responsible AI training modules (Machine Learning University)
- What traps can we fall into when modeling a social problem?
- Alan F. Winfield ; Katina Michael ; Jeremy Pitt ; Vanessa Evers: Machine Ethics: The Design and Governance of Ethical AI and Autonomous Systems
- Responsible AI training modules (Machine Learning University)
- Artificial Intelligence and Data Analytics (AIDA) Guidebook
- Responsible AI from principles to practice - Virginia Dignum